해당 내용은 Andrew Ng 교수님의 Machine Learning 강의(Coursera)를 정리한 내용입니다.
※ 아래에 문제 풀이가 있습니다. 원하지 않는다면 스크롤을 내리지 마세요.
5주차 과제는 다음과 같다.
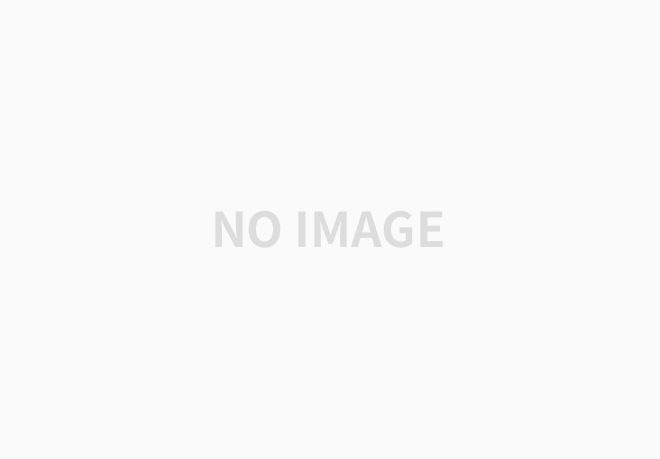
sigmoidGradient.m - sigmoid function의 미분을 계산하는 코드
randInitializeWeights.m - Parameter 을 random 값으로 초기화하는 코드
nnCostFunction.m - Neural Network의 Cost Function 코드
https://github.com/junstar92/Coursera/tree/master/MachineLearning/ex4
[sigmoidGradient.m]
sigmoid function은 로 나타낼 수 있다. 그리고 미분을 하면 다음과 같다.
미분 방법은 다음 글에 설명이 되어 있다.
그래서 코드로 작성하면 다음과 같다.
function g = sigmoidGradient(z) %SIGMOIDGRADIENT returns the gradient of the sigmoid function %evaluated at z % g = SIGMOIDGRADIENT(z) computes the gradient of the sigmoid function % evaluated at z. This should work regardless if z is a matrix or a % vector. In particular, if z is a vector or matrix, you should return % the gradient for each element. g = zeros(size(z)); % ====================== YOUR CODE HERE ====================== % Instructions: Compute the gradient of the sigmoid function evaluated at % each value of z (z can be a matrix, vector or scalar). g = sigmoid(z).*(1 - sigmoid(z)); % ============================================================= end
[randInitializeWeights.m]
Neural Network에서 Parameter 값을 초기화를 해주어야 한다. 이때, 0으로 초기화를 하게 되면 적절하지 않기 때문에 랜덤한 값으로 초기화를 해야하며, 자세한 내용은 아래 글의 Random Initialization 부분을 참조하기 바란다.
코드는 다음과 같다. 의 값으로 0.12를 사용하였고, 이는 ex4.pdf의 가이드를 참조했다)
function W = randInitializeWeights(L_in, L_out) %RANDINITIALIZEWEIGHTS Randomly initialize the weights of a layer with L_in %incoming connections and L_out outgoing connections % W = RANDINITIALIZEWEIGHTS(L_in, L_out) randomly initializes the weights % of a layer with L_in incoming connections and L_out outgoing % connections. % % Note that W should be set to a matrix of size(L_out, 1 + L_in) as % the first column of W handles the "bias" terms % % You need to return the following variables correctly W = zeros(L_out, 1 + L_in); % ====================== YOUR CODE HERE ====================== % Instructions: Initialize W randomly so that we break the symmetry while % training the neural network. % % Note: The first column of W corresponds to the parameters for the bias unit % EPSILON = 0.12; W = rand(L_out, L_in + 1) * (2 * EPSILON) - EPSILON; % ========================================================================= end
[nnCostFunction.m]
NN의 CostFunction 코드를 다음의 순서로 동작하도록 작성해야 한다.
1. FP(Forward Propagation)을 진행하고 Cost Function J의 값을 구한다.
2. BP(Back-Propagation)을 진행하고, 를 구한다. 그리고 Gradient Checking을 한다.(checkNNGradient 함수를 통해)
3. CostFunction과 Gradient를 가지고 Regularizatoin 한다.
전체 코드는 다음과 같다.
function [J grad] = nnCostFunction(nn_params, ... input_layer_size, ... hidden_layer_size, ... num_labels, ... X, y, lambda) %NNCOSTFUNCTION Implements the neural network cost function for a two layer %neural network which performs classification % [J grad] = NNCOSTFUNCTON(nn_params, hidden_layer_size, num_labels, ... % X, y, lambda) computes the cost and gradient of the neural network. The % parameters for the neural network are "unrolled" into the vector % nn_params and need to be converted back into the weight matrices. % % The returned parameter grad should be a "unrolled" vector of the % partial derivatives of the neural network. % % Reshape nn_params back into the parameters Theta1 and Theta2, the weight matrices % for our 2 layer neural network Theta1 = reshape(nn_params(1:hidden_layer_size * (input_layer_size + 1)), ... hidden_layer_size, (input_layer_size + 1)); Theta2 = reshape(nn_params((1 + (hidden_layer_size * (input_layer_size + 1))):end), ... num_labels, (hidden_layer_size + 1)); % Setup some useful variables m = size(X, 1); % You need to return the following variables correctly J = 0; Theta1_grad = zeros(size(Theta1)); Theta2_grad = zeros(size(Theta2)); % ====================== YOUR CODE HERE ====================== % Instructions: You should complete the code by working through the % following parts. % % Part 1: Feedforward the neural network and return the cost in the % variable J. After implementing Part 1, you can verify that your % cost function computation is correct by verifying the cost % computed in ex4.m % % Part 2: Implement the backpropagation algorithm to compute the gradients % Theta1_grad and Theta2_grad. You should return the partial derivatives of % the cost function with respect to Theta1 and Theta2 in Theta1_grad and % Theta2_grad, respectively. After implementing Part 2, you can check % that your implementation is correct by running checkNNGradients % % Note: The vector y passed into the function is a vector of labels % containing values from 1..K. You need to map this vector into a % binary vector of 1's and 0's to be used with the neural network % cost function. % % Hint: We recommend implementing backpropagation using a for-loop % over the training examples if you are implementing it for the % first time. % % Part 3: Implement regularization with the cost function and gradients. % % Hint: You can implement this around the code for % backpropagation. That is, you can compute the gradients for % the regularization separately and then add them to Theta1_grad % and Theta2_grad from Part 2. % % Setting Y matrix to m(5000) x classes(10) Y = zeros(m, num_labels); for i = 1:m Y(i, y(i)) = 1; end % ----------------- Part 1 : FP ------------------------------- a1 = [ones(m, 1) X]; % a1 = 5000 x 401 z2 = a1 * Theta1'; % a1(5000 x 401) x Theta1'(401 x 25) = z2(5000 x 25) a2 = zeros(m, hidden_layer_size); a2 = [ones(m, 1), sigmoid(a1*Theta1')]; % a2 = 5000 x 26 z3 = a2 * Theta2'; % a2(5000 x 26) x Theta2'(26 x 10) = z3(5000 x 10) a3 = sigmoid(z3); % a3 = 5000 x 10 % Y = 5000 x 10, a3 = 5000 x 10 J = -(1/m)*(sum(sum(Y.*log(a3) + (1-Y).*log(1 - a3)))) ... + (lambda / (2*m)) * (sum(sum(Theta1(:,2:end).^2)) + sum(sum(Theta2(:,2:end).^2))); % ----------------- Part 2, 3 : BP ----------------------------- d3 = (a3 - Y); % delta3 = 5000 x 10 d2 = (Theta2(:, 2:end)' * d3') .* sigmoidGradient(z2)'; % Theta2(except for column 1, 10 x 25) x delta3' = delta2(25 x 5000) D1 = d2 * a1; % Delta1(25 x 401) = delta2(25 x 5000) x a1(5000 x 401) D2 = d3' * a2; % Delta2(10 x 26) = delta3'(10 x 5000) x a2(5000 x 26) reg1 = lambda * [zeros(size(Theta1, 1), 1) Theta1(:,2:end)]; reg2 = lambda * [zeros(size(Theta2, 1), 1) Theta2(:,2:end)]; Theta1_grad = (1/m)*(D1 + reg1); % 25 x 401 Theta2_grad = (1/m)*(D2 + reg2); % 10 x 26 % ------------------------------------------------------------- % ========================================================================= % Unroll gradients grad = [Theta1_grad(:) ; Theta2_grad(:)]; end
[line 66 ~ 69]
현재 주어진 Training Set의 결과값 y는 5000 x 1의 행렬로 Training Set에 대한 label(Class)이다. 우리가 계산한 Hypothesis Function의 결과는 각 Class에 대한 확률값으로 나타나기 때문에 최종 Hypothesis 값은 m(5000) \times classes(10)의 행렬로 나타난다. 그래서 첫 번째로 우리는 현재 y의 결과를 각 클래스 별 확률값으로 변환해주어야 하고, 그 확률값은 Training Set의 input에 대한 output class에 해당하는 열만 1이고 나머지 열은 0으로 대입하면 된다.
[line 71 ~ 81 : FP]
FP의 과정이다.
우리는 Input으로부터 를 구하고, 최종적으로 이 된다. 결과는 각 Class의 확률값으로 나타나기 때문에 이 된다.
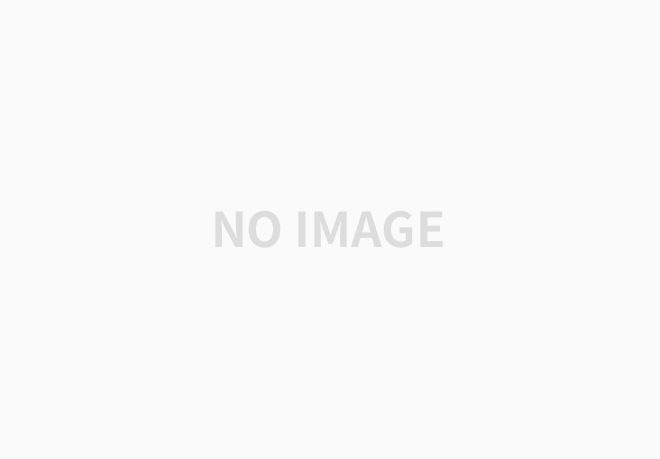
그리고 Cost Function의 값을 위의 식으로부터 구한다(Line 80).
[line 83 ~ 94 : BP]
다음은 BP의 과정이다. 앞서 우리는 FP의 과정을 진행했기 때문에, FP한 결과를 바탕으로 BP의 나머지 부분을 진행하면 된다.
여기서 최종적으로 구하게 되는 는 결국 에 대한 편미분이므로, 의 차원으로 나타나게 된다. 즉, 위에서 우리가 최종적으로 구해야하는 Theta1_grad와 Theta2_grad가 Theta1과 Theta2의 차원과 동일하다고 먼저 알고 있으면 행렬을 계산하는데 도움이 된다.
어떻게 Transformation에 하느냐에 따라 식이 조금 바뀔 수 있지만, 나는 FP에서 구한 요소들(a1, a2)를 그대로 사용하려고 위와 같이 구현했다. 코드 우측에 행렬의 차원을 주석으로 달아놓아서 참고하면 되겠다.
아래와 같은 식을 코드로 구현한 것이다.
(line 84)
(line 86)
(line 87)
(line 88)
그리고, 추가적으로 아래와 같이 정규화항을 더해주어야 한다.
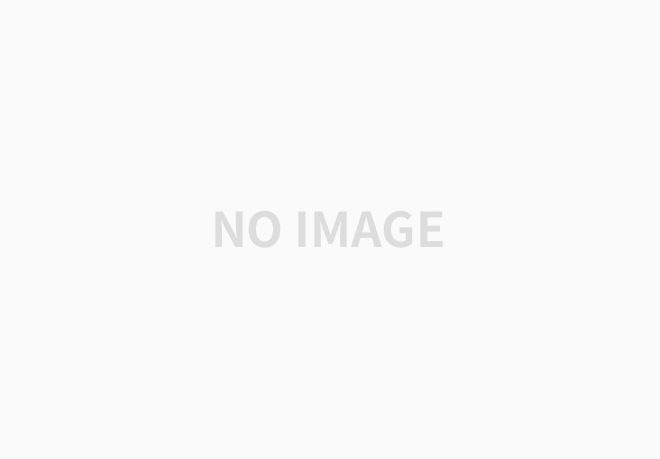
정규화항이 line90, 91에 해당하고 나는 우선 을 구하였다.
그리고, (line 93, 94)로 를 구하였다.
'Coursera 강의 > Machine Learning' 카테고리의 다른 글
[Machine Learning] Exam 5 (Week 6) (0) | 2020.08.19 |
---|---|
[Machine Learning] Advice for Applying Machine Learning 1 (0) | 2020.08.18 |
[Machine Learning] Backpropagation in Practice (0) | 2020.08.15 |
[Machine Learning] Neural Network(Cost Function, Backpropagation Algorithm) (0) | 2020.08.15 |
[Machine Learning] Exam 3 (Week 4) (0) | 2020.08.14 |
댓글