해당 내용은 Coursera의 딥러닝 특화과정(Deep Learning Specialization)의 두 번째 강의
Improving Deep Neural Networks: Hyperparameter tuning, Regularization and Optimization를 듣고 정리한 내용입니다. (Week 1)
1주차 두 번째 실습은 Regularization 입니다.
딥러닝 모델은 매우 높은 flexibility와 capacity를 가지고 있어서, dataset이 충분히 크기 않다면 overfitting하는 심각한 문제를 일으킬 수 있습니다. overfitting은 training set에는 잘 맞지만, 새로운 sample에 대해서는 일반화되지 않는 문제를 일으킵니다.
이번 실습에서 regularization을 사용해서 딥러닝 모델을 학습해봅시다.
사용되는 패키지는 다음과 같습니다.
# import packages import numpy as np import matplotlib.pyplot as plt from reg_utils import sigmoid, relu, plot_decision_boundary, initialize_parameters, load_2D_dataset, predict_dec from reg_utils import compute_cost, predict, forward_propagation, backward_propagation, update_parameters import sklearn import sklearn.datasets import scipy.io from testCases import * %matplotlib inline plt.rcParams['figure.figsize'] = (7.0, 4.0) # set default size of plots plt.rcParams['image.interpolation'] = 'nearest' plt.rcParams['image.cmap'] = 'gray'
예측하려는 문제는 골키퍼가 공을 찾 때, 어디로 차야지 같은 팀 선수가 머리로 공을 받을 수 있는지 예측하는 것입니다.
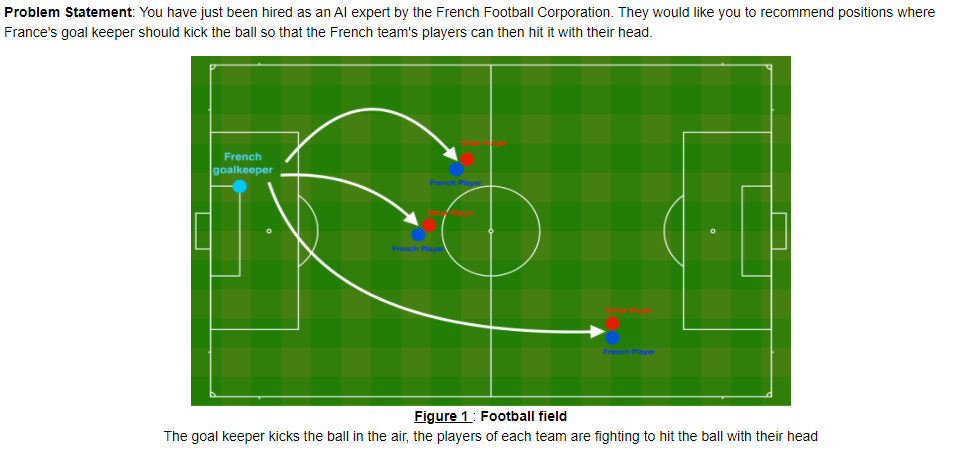
입력은 2차원의 dataset으로 주어지며, 과거 10 게임의 결과입니다.

blue point는 같은 팀(French) 선수가 머리로 공을 받은 것을 의미하며, red point는 다른팀 선수가 머리로 공을 받은 것을 의미합니다.
데이터를 살펴보면, 조금 noisy하긴 하지만, 대각선 왼쪽 위로 공을 찼을 때, 같은 팀 선수가 잘 받는 것으로 보입니다.
첫 번째로는 regularization을 적용하지 않은 모델로 시도해보겠습니다. 그리고, regularization을 사용해서 문제를 예측해보도록 하겠습니다.
1. Non-regularized model
아래와 같은 model 함수를 사용하겠습니다.
이 함수는 regularization mode와 dropout mode로도 사용될 수 있는데,
- regularization mode에서는 lambd 값은 0이 아닌 값으로 설정하면 되고,
- dropout mode에서는 keep_prob 값을 1보다 낮은 값으로 설정하면 됩니다.
def model(X, Y, learning_rate = 0.3, num_iterations = 30000, print_cost = True, lambd = 0, keep_prob = 1): """ Implements a three-layer neural network: LINEAR->RELU->LINEAR->RELU->LINEAR->SIGMOID. Arguments: X -- input data, of shape (input size, number of examples) Y -- true "label" vector (1 for blue dot / 0 for red dot), of shape (output size, number of examples) learning_rate -- learning rate of the optimization num_iterations -- number of iterations of the optimization loop print_cost -- If True, print the cost every 10000 iterations lambd -- regularization hyperparameter, scalar keep_prob - probability of keeping a neuron active during drop-out, scalar. Returns: parameters -- parameters learned by the model. They can then be used to predict. """ grads = {} costs = [] # to keep track of the cost m = X.shape[1] # number of examples layers_dims = [X.shape[0], 20, 3, 1] # Initialize parameters dictionary. parameters = initialize_parameters(layers_dims) # Loop (gradient descent) for i in range(0, num_iterations): # Forward propagation: LINEAR -> RELU -> LINEAR -> RELU -> LINEAR -> SIGMOID. if keep_prob == 1: a3, cache = forward_propagation(X, parameters) elif keep_prob < 1: a3, cache = forward_propagation_with_dropout(X, parameters, keep_prob) # Cost function if lambd == 0: cost = compute_cost(a3, Y) else: cost = compute_cost_with_regularization(a3, Y, parameters, lambd) # Backward propagation. assert(lambd==0 or keep_prob==1) # it is possible to use both L2 regularization and dropout, # but this assignment will only explore one at a time if lambd == 0 and keep_prob == 1: grads = backward_propagation(X, Y, cache) elif lambd != 0: grads = backward_propagation_with_regularization(X, Y, cache, lambd) elif keep_prob < 1: grads = backward_propagation_with_dropout(X, Y, cache, keep_prob) # Update parameters. parameters = update_parameters(parameters, grads, learning_rate) # Print the loss every 10000 iterations if print_cost and i % 10000 == 0: print("Cost after iteration {}: {}".format(i, cost)) if print_cost and i % 1000 == 0: costs.append(cost) # plot the cost plt.plot(costs) plt.ylabel('cost') plt.xlabel('iterations (x1,000)') plt.title("Learning rate =" + str(learning_rate)) plt.show() return parameters
우선 우리는 regularization없이 모델을 학습해보겠습니다.
parameters = model(train_X, train_Y) print ("On the training set:") predictions_train = predict(train_X, train_Y, parameters) print ("On the test set:") predictions_test = predict(test_X, test_Y, parameters)
보다시피 lambd와 keep_prob값을 설정하지 않았기 때문에, 기본값으로 설정되어 regularization이 적용되지 않고 학습하게 됩니다.
결과는 다음과 같습니다.

traininig set의 정확도는 약 94.8%이고, test set의 정확도는 91.5% 입니다. 이 값을 기준으로 regularization의 효과를 비교해보도록 하겠습니다.
non-regularization의 Decision Boundary는 다음과 같이 나타납니다.

non-regularized model은 명백히 training set에 overfitting되어 보입니다.(파란색 영역에 빨간 영역이 있거나, 빨간색 영역에 파란색 영역이 있는 것으로 보아)
이제 regularization으로 overfitting을 감소시켜 봅시다.
2. L2 Regularization
overfitting을 피하는 기본적인 방법은 L2 Regularization 입니다.
L2 Regularization을 적용한 Cost Function은 다음과 같습니다.

compute_cost_with_regularization 함수를 통해서 위 식의 Cost를 구합니다. regularization항은 np.sum(np.sqaure(Wl))로 구하면 됩니다.
# GRADED FUNCTION: compute_cost_with_regularization def compute_cost_with_regularization(A3, Y, parameters, lambd): """ Implement the cost function with L2 regularization. See formula (2) above. Arguments: A3 -- post-activation, output of forward propagation, of shape (output size, number of examples) Y -- "true" labels vector, of shape (output size, number of examples) parameters -- python dictionary containing parameters of the model Returns: cost - value of the regularized loss function (formula (2)) """ m = Y.shape[1] W1 = parameters["W1"] W2 = parameters["W2"] W3 = parameters["W3"] cross_entropy_cost = compute_cost(A3, Y) # This gives you the cross-entropy part of the cost ### START CODE HERE ### (approx. 1 line) L2_regularization_cost = lambd/(2*m)*(np.sum(np.square(W1)) + np.sum(np.square(W2)) + np.sum(np.square(W3))) ### END CODER HERE ### cost = cross_entropy_cost + L2_regularization_cost return cost
마찬가지로 BP 또한 regularization이 적용되어야 합니다. cost가 변경되었기 때문에 모든 gradient도 새로운 cost에 대해서 구해집니다. regularization term의 gradient는 다음과 같습니다.
\[\frac{d}{dW}(\frac{1}{2}\frac{\lambda}{m}W^{2}) = \frac{\lambda}{m}W\]
# GRADED FUNCTION: backward_propagation_with_regularization def backward_propagation_with_regularization(X, Y, cache, lambd): """ Implements the backward propagation of our baseline model to which we added an L2 regularization. Arguments: X -- input dataset, of shape (input size, number of examples) Y -- "true" labels vector, of shape (output size, number of examples) cache -- cache output from forward_propagation() lambd -- regularization hyperparameter, scalar Returns: gradients -- A dictionary with the gradients with respect to each parameter, activation and pre-activation variables """ m = X.shape[1] (Z1, A1, W1, b1, Z2, A2, W2, b2, Z3, A3, W3, b3) = cache dZ3 = A3 - Y ### START CODE HERE ### (approx. 1 line) dW3 = 1./m * np.dot(dZ3, A2.T) + lambd/m * W3 ### END CODE HERE ### db3 = 1./m * np.sum(dZ3, axis=1, keepdims = True) dA2 = np.dot(W3.T, dZ3) dZ2 = np.multiply(dA2, np.int64(A2 > 0)) ### START CODE HERE ### (approx. 1 line) dW2 = 1./m * np.dot(dZ2, A1.T) + lambd/m * W2 ### END CODE HERE ### db2 = 1./m * np.sum(dZ2, axis=1, keepdims = True) dA1 = np.dot(W2.T, dZ2) dZ1 = np.multiply(dA1, np.int64(A1 > 0)) ### START CODE HERE ### (approx. 1 line) dW1 = 1./m * np.dot(dZ1, X.T) + lambd/m * W1 ### END CODE HERE ### db1 = 1./m * np.sum(dZ1, axis=1, keepdims = True) gradients = {"dZ3": dZ3, "dW3": dW3, "db3": db3,"dA2": dA2, "dZ2": dZ2, "dW2": dW2, "db2": db2, "dA1": dA1, "dZ1": dZ1, "dW1": dW1, "db1": db1} return gradients
\(\lambda = 0.7\)인 L2 regularization이 적용된 모델을 학습해보겠습니다.
parameters = model(train_X, train_Y, lambd = 0.7) print ("On the train set:") predictions_train = predict(train_X, train_Y, parameters) print ("On the test set:") predictions_test = predict(test_X, test_Y, parameters)
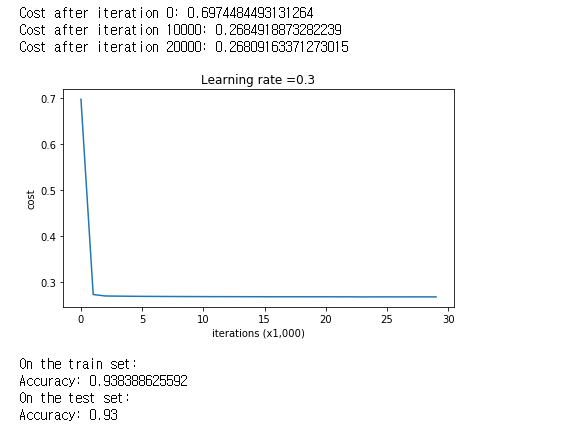
test set의 정확도가 93%로 증가한 것을 볼 수 있습니다.
Decision Boundary는 다음과 같이 그려집니다.

꽤 일반화가 잘되어서 경계가 나누어졌습니다.
여기서 \(\lambda\)는 dev set을 통해서 튜닝해야하는 hyperparameter입니다.
What you should remember -- the implications of L2-regularization on:
- The cost computation:
- A regularization term is added to the cost
- The backpropagation function:
- There are extra terms in the gradients with respect to weight matrices
- Weights end up smaller ("weight decay"):
- Weights are pushed to smaller values.
3. Dropout
마지막으로 살펴볼 dropout은 딥러닝에 특화되는 regularization 기법입니다.
dropout은 매 iteration마다 랜덤하게 몇몇의 neuron을 제거합니다.
이때, 1 - keep_prob의 확률로 layer의 neuron을 제거(0으로 만듦)하고, keep_prob의 확률로 유지합니다. 제거된 neuron은 학습(FP와 BP)에서 제외됩니다.
이렇게 일부 neuron이 제거되면, 특정 neuron이 언제든지 제거될 수 있으므로, 결과값이 특정 neuron에 민감해지지 않도록 합니다.
3.1 Forward propagation with dropout
3-layer NN을 사용해서 dropout을 첫 번째와 두 번째 hidden layer에 적용해보겠습니다. 보통 input과 output layer에는 dropout을 적용하지 않습니다.
dropout은 다음과 같은 과정을 통해서 진행합니다.
1. np.random.rand()를 사용해서 \(a^{[1]}\)과 같은 shape를 갖는 \(d^{[1]}\)를 생성하는데, 랜덤 행렬인 \(D^{[1]} = \begin{bmatrix} d^{[1](1)} && d^{[1](2)} && \cdots && d^{[1](m)} \end{bmatrix}\)를 만들면 됩니다. \(D^{[1]}\)은 \(A^{[1]}\)과 동일한 차원입니다.
2. \(D^{[1]}\)의 각 요소를 keep_prob 확률에 따라서 1과 0으로 설정합니다.
이때, X = (X < keep_prob).astype(int) 를 통해서 반복문없이 쉽게 1과 0으로 변환할 수 있습니다.
3. \(A^{[1]}\)의 값을 \(A^{[1]} \ast D^{[1]}\)로 변환합니다. 즉, \(D^{[1]}\)은 mask로 사용하고, 몇몇의 neuron이 0으로 설정될 것입니다.
4. 이렇게 구한 \(A^{[1]}\) 값을 keep_prob 값으로 나누어 줍니다. 이것은 cost의 결과가 dropout없이 계산한 cost의 exlpected value와 같도록 합니다. 이 기법은 inverted dropout으로 불립니다.
# GRADED FUNCTION: forward_propagation_with_dropout def forward_propagation_with_dropout(X, parameters, keep_prob = 0.5): """ Implements the forward propagation: LINEAR -> RELU + DROPOUT -> LINEAR -> RELU + DROPOUT -> LINEAR -> SIGMOID. Arguments: X -- input dataset, of shape (2, number of examples) parameters -- python dictionary containing your parameters "W1", "b1", "W2", "b2", "W3", "b3": W1 -- weight matrix of shape (20, 2) b1 -- bias vector of shape (20, 1) W2 -- weight matrix of shape (3, 20) b2 -- bias vector of shape (3, 1) W3 -- weight matrix of shape (1, 3) b3 -- bias vector of shape (1, 1) keep_prob - probability of keeping a neuron active during drop-out, scalar Returns: A3 -- last activation value, output of the forward propagation, of shape (1,1) cache -- tuple, information stored for computing the backward propagation """ np.random.seed(1) # retrieve parameters W1 = parameters["W1"] b1 = parameters["b1"] W2 = parameters["W2"] b2 = parameters["b2"] W3 = parameters["W3"] b3 = parameters["b3"] # LINEAR -> RELU -> LINEAR -> RELU -> LINEAR -> SIGMOID Z1 = np.dot(W1, X) + b1 A1 = relu(Z1) ### START CODE HERE ### (approx. 4 lines) # Steps 1-4 below correspond to the Steps 1-4 described above. D1 = np.random.rand(A1.shape[0], A1.shape[1]) # Step 1: initialize matrix D1 = np.random.rand(..., ...) D1 = (D1 < keep_prob).astype(int) # Step 2: convert entries of D1 to 0 or 1 (using keep_prob as the threshold) A1 = np.multiply(A1, D1) # Step 3: shut down some neurons of A1 A1 = A1 / keep_prob # Step 4: scale the value of neurons that haven't been shut down ### END CODE HERE ### Z2 = np.dot(W2, A1) + b2 A2 = relu(Z2) ### START CODE HERE ### (approx. 4 lines) D2 = np.random.rand(A2.shape[0], A2.shape[1]) # Step 1: initialize matrix D2 = np.random.rand(..., ...) D2 = (D2 < keep_prob).astype(int) # Step 2: convert entries of D2 to 0 or 1 (using keep_prob as the threshold) A2 = np.multiply(A2, D2) # Step 3: shut down some neurons of A2 A2 = A2 / keep_prob # Step 4: scale the value of neurons that haven't been shut down ### END CODE HERE ### Z3 = np.dot(W3, A2) + b3 A3 = sigmoid(Z3) cache = (Z1, D1, A1, W1, b1, Z2, D2, A2, W2, b2, Z3, A3, W3, b3) return A3, cache
1,2 - layer에서만 dropout을 적용합니다.
3.2 Backward propagation with dropout
dropout이 적용된 BP는 꽤 심플하고, 단순히 2단계로 진행됩니다.
1. FP에서 구한 mask \(D^{[1]}\)를 A1에 적용합니다. BP에서도 동일한 neuron을 제거하며, FP에서 사용한 \(D^{[1]}\)를 A1에 재적용합니다.
2. FP에서 A1을 keep_prob로 나누어준 것처럼, BP에서도 동일하게 dA1을 keep_prob로 나누어줍니다. 미적분학 해석으로 \(A^{[1]}\)이 keep_prob에 의해서 scaling되었으면, 그의 미분항 \(dA^{[1]}\)도 keep_prob로 scaling 됩니다.
# GRADED FUNCTION: backward_propagation_with_dropout def backward_propagation_with_dropout(X, Y, cache, keep_prob): """ Implements the backward propagation of our baseline model to which we added dropout. Arguments: X -- input dataset, of shape (2, number of examples) Y -- "true" labels vector, of shape (output size, number of examples) cache -- cache output from forward_propagation_with_dropout() keep_prob - probability of keeping a neuron active during drop-out, scalar Returns: gradients -- A dictionary with the gradients with respect to each parameter, activation and pre-activation variables """ m = X.shape[1] (Z1, D1, A1, W1, b1, Z2, D2, A2, W2, b2, Z3, A3, W3, b3) = cache dZ3 = A3 - Y dW3 = 1./m * np.dot(dZ3, A2.T) db3 = 1./m * np.sum(dZ3, axis=1, keepdims = True) dA2 = np.dot(W3.T, dZ3) ### START CODE HERE ### (≈ 2 lines of code) dA2 = np.multiply(dA2, D2) # Step 1: Apply mask D2 to shut down the same neurons as during the forward propagation dA2 = dA2 / keep_prob # Step 2: Scale the value of neurons that haven't been shut down ### END CODE HERE ### dZ2 = np.multiply(dA2, np.int64(A2 > 0)) dW2 = 1./m * np.dot(dZ2, A1.T) db2 = 1./m * np.sum(dZ2, axis=1, keepdims = True) dA1 = np.dot(W2.T, dZ2) ### START CODE HERE ### (≈ 2 lines of code) dA1 = np.multiply(dA1, D1) # Step 1: Apply mask D1 to shut down the same neurons as during the forward propagation dA1 = dA1 / keep_prob # Step 2: Scale the value of neurons that haven't been shut down ### END CODE HERE ### dZ1 = np.multiply(dA1, np.int64(A1 > 0)) dW1 = 1./m * np.dot(dZ1, X.T) db1 = 1./m * np.sum(dZ1, axis=1, keepdims = True) gradients = {"dZ3": dZ3, "dW3": dW3, "db3": db3,"dA2": dA2, "dZ2": dZ2, "dW2": dW2, "db2": db2, "dA1": dA1, "dZ1": dZ1, "dW1": dW1, "db1": db1} return gradients
이렇게 구현한 함수로 keep_prob가 0.86으로 설정해서 3-layer NN을 학습해보겠습니다.
parameters = model(train_X, train_Y, keep_prob = 0.86, learning_rate = 0.3) print ("On the train set:") predictions_train = predict(train_X, train_Y, parameters) print ("On the test set:") predictions_test = predict(test_X, test_Y, parameters)

dropout이 아주 잘 동작합니다. test set의 정확도는 95%까지 증가했습니다. 모델은 더이상 training set에 overfitting하지 않고, test set에도 잘 맞습니다.
Decision Boundary는 다음과 같이 그려집니다.
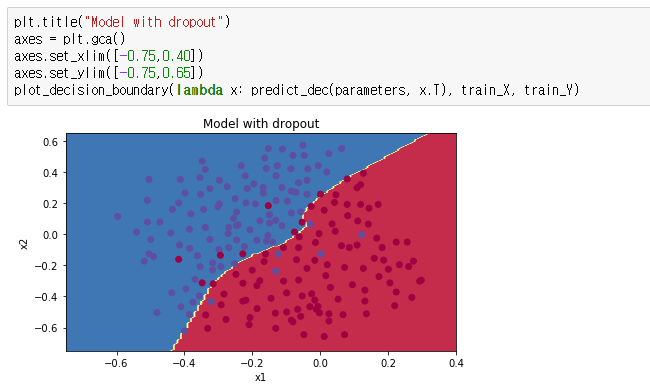
주의해야할 점은 dropout은 오직 training할 때만 적용해야하며, test set에는 사용하면 안됩니다.
딥러닝 framework인 tensorflow, PaddelPaddle, Keras or caffe에서는 dropout이 제공되므로, 추후에 이러한 framework를 배워보도록 하겠습니다.
What you should remember about dropout:
- Dropout is a regularization technique.
- You only use dropout during training. Don't use dropout (randomly eliminate nodes) during test time.
- Apply dropout both during forward and backward propagation.
- During training time, divide each dropout layer by keep_prob to keep the same expected value for the activations. For example, if keep_prob is 0.5, then we will on average shut down half the nodes, so the output will be scaled by 0.5 since only the remaining half are contributing to the solution. Dividing by 0.5 is equivalent to multiplying by 2. Hence, the output now has the same expected value. You can check that this works even when keep_prob is other values than 0.5.
4. Conclusions
3가지 방법을 비교하면 다음과 같습니다.
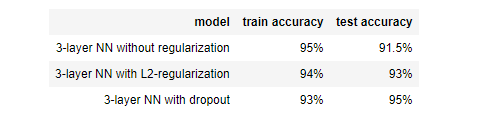
- Regularization은 overfitting을 감소하는데 도움이 됩니다.
- Regularization은 파라미터 W의 값을 낮추어 줍니다.
- L2 Regularization과 Dropout은 매우 효과적인 regularization 기법입니다.
지금까지 regularization의 효과를 살펴보았습니다.
'Coursera 강의 > Deep Learning' 카테고리의 다른 글
Optimization(최적화 알고리즘) : Mini-batch/Momentum/RMSprop/Adam (0) | 2020.10.02 |
---|---|
[실습] Gradient Checking (0) | 2020.09.26 |
[실습] Initialization 초기화 (0) | 2020.09.26 |
[실습] Deep Neural Network for Image Classification(cat vs non-cat) (6) | 2020.09.26 |
[실습] Building Deep Neural Network : Step by Step (0) | 2020.09.25 |
댓글