(tensorflow v2.4.0)
Functional API와 Sequential API를 사용해서 여러개의 input이나 여러개의 output을 가지는 Model을 구성할 수 있습니다.
아래는 그 방법으로 구성한 Wide and Deep model 입니다.
import tensorflow as tf from tensorflow.keras.utils import plot_model from tensorflow.keras.layers import Input, Dense, concatenate from tensorflow.keras.models import Model # functional API # define inputs input_a = Input(shape=[1], name="Wide_Input") input_b = Input(shape=[1], name="Deep_Input") # define deep path hidden_1 = Dense(30, activation="relu")(input_b) hidden_2 = Dense(30, activation="relu")(hidden_1) # define merged path concat = concatenate([input_a, hidden_2]) output = Dense(1, name="Output")(concat) # define another output for the deep path aux_output = Dense(1,name="aux_Output")(hidden_2) # build the model model = Model(inputs=[input_a, input_b], outputs=[output, aux_output]) # visualize the architecture plot_model(model)
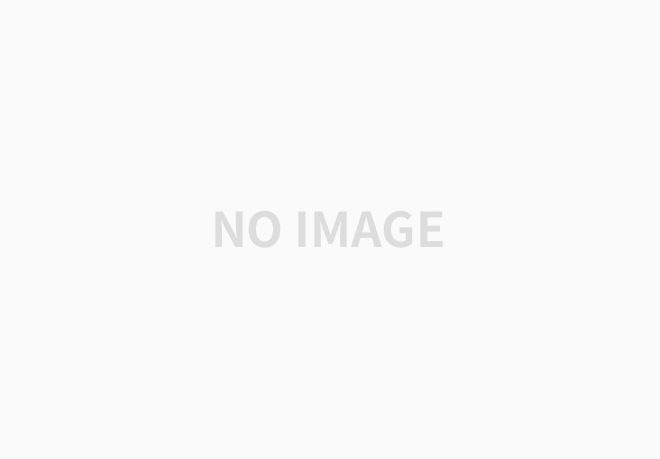
하지만, 이 방법만 존재하는 것이 아닙니다.
tensorflow에서는 Model class를 상속하여서, 자시만의 모델을 구성할 수 있습니다.
일단 어떻게 구현하는지 살펴보도록 합시다.
# encapsuling into a class class WideAndDeepModel(Model): def __init__(self, units=30, activation='relu', **kwargs): super(WideAndDeepModel, self).__init__() self.hidden1 = Dense(units, activation=activation) self.hidden2 = Dense(units, activation=activation) self.main_output = Dense(1) self.aux_output = Dense(1) def call(self, inputs): input_a, input_b = inputs hidden1 = self.hidden1(input_b) hidden2 = self.hidden2(hidden2) concat = concatenate([input_a, hidden2]) main_output = self.main_output(concat) aux_output = self.aux_output(hidden2) return main_output, aux_output
tf.keras.models.Model Class를 상속받아서 정의할 수 있으며, custom layer를 Layer class를 통해서 구성하는 것과 동일하게, __init__ 함수를 통해서 Model Class의 속성을 상속받고 call 함수를 오버라이딩해서 모델의 computation을 정의합니다.
이렇게 생성된 모델은 mode = WideAndDeepModel() 문법을 통해서 사용할 수 있게 됩니다.
Using the Model class
model class를 사용한 model은 Sequential model 또는 Functional APIs model 처럼 아래의 공통점이 있습니다.
공통점
- Built-in training, evaluation, prediction loops를 사용할 수 있음(e.g., model.fit(), model.evaluate(), model.predict())
- Saving and serialization APIs (e.g., model.save(), model.save_weights())
- Summarization and visualization APIs (e.g., model.summary(), tf.keras.utils.plot_model())
Model Class에서 제공하는 method를 사용할 수 있다는 것이죠.
그리고, Sequential model과 Functional APIs model은 불가능한 cycle graphs layer를 구성하거나(ex, MobileNet, Inception), 더 특이한 구조의 모델(dynamic and recursive networks)을 구성할 수 있습니다.
즉, Sequential model과 Functional APIs는 Directed Acyclic Graphs의 layer로만 모델을 구성할 수 있는데, Model Class를 사용한 네트워크는 그렇지 않다는 것입니다.
그래서 Model Class를 상속한 네트워크는 다음과 같은 이점을 갖고 있습니다.
- Extends how you've been building models
- Continue to use functional and sequential code
- Modular architecture
- Try out experiments quickly
- Control flow in the network
이러한 장점들을 기억하면서, ResNet18의 일부분을 떼와서 Mini-ResNet과 VGGNet을 직접 구현해보도록 하겠습니다.
ResNet과 VGG 모델의 설명은 제가 딥러닝 특화과정 수업을 통해 정리한 내용을 참조하시거나, 검색을 통해서 살펴보시기 바랍니다.. !
Mini ResNet
ResNet-18 모델은 Identity Block과 Identity Block with 1x1 Convolution 들로 구성되어 있습니다.
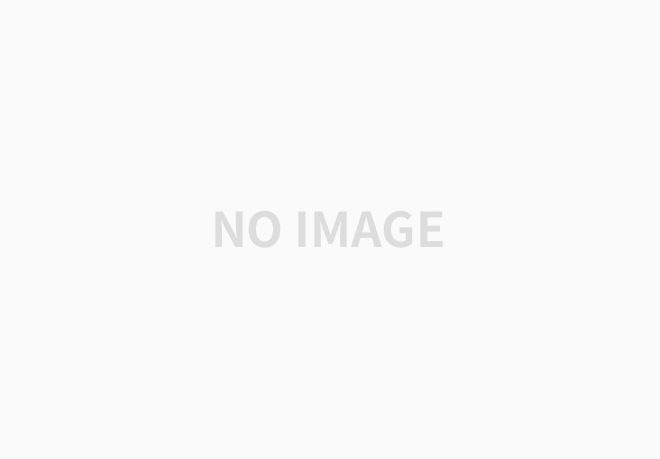
우리는 Identity Block만을 가져와서 Mini-ResNet을 구현할 예정입니다. 중간에 파란색 layer들이 Identity Block이며, 총 2개가 있습니다.
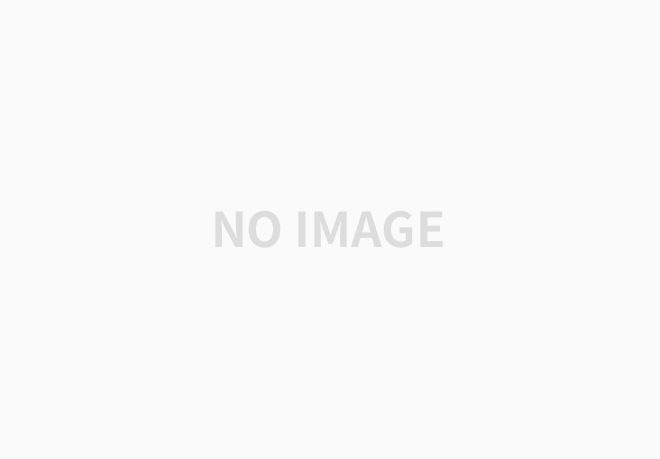
우선 Identity Block을 구현해보도록 합시다.
# Identity Block class IdentityBlock(tf.keras.Model): def __init__(self, filters, kernel_size): super(IdentityBlock, self).__init__(name='') self.conv1 = tf.keras.layers.Conv2D(filters, kernel_size, padding='same') self.bn1 = tf.keras.layers.BatchNormalization() self.conv2 = tf.keras.layers.Conv2D(filters, kernel_size, padding='same') self.bn2 = tf.keras.layers.BatchNormalization() self.relu = tf.keras.layers.Activation('relu') self.add = tf.keras.layers.Add() def call(self, inputs): x = self.conv1(inputs) x = self.bn1(x) x = self.relu(x) x = self.conv2(x) x = self.bn2(x) x = self.add([x, inputs]) x = self.relu(x) return x
__init__ 함수에서 사용될 layer들을 정의해주고, call 함수를 통해서 computation을 구현합니다. 계산 마지막 즈음에 inputs이 더해져서 출력이 되고 있는 것을 확인할 수 있습니다.
이제 Mini ResNet 모델을 구성해보도록 하겠습니다.
class ResNet(tf.keras.Model): def __init__(self, num_classes): super(ResNet, self).__init__() self.conv = tf.keras.layers.Conv2D(64, 7, padding='same') self.bn = tf.keras.layers.BatchNormalization() self.relu = tf.keras.layers.Activation('relu') self.max_pool = tf.keras.layers.MaxPool2D((3, 3)) self.id1a = IdentityBlock(64, 3) self.id1b = IdentityBlock(64, 3) self.global_pool = tf.keras.layers.GlobalAveragePooling2D() self.classifier = tf.keras.layers.Dense(num_classes, activation='softmax') def call(self, inputs): x = self.conv(inputs) x = self.bn(x) x = self.relu(x) x = self.max_pool(x) x = self.id1a(x) x = self.id1b(x) x = self.global_pool(x) return self.classifier(x)
구현된 Mini ResNet입니다. 마지막 classifier를 통해 파라미터로 입력받은 num_classes의 개수만큼 softmax 확률 결과를 출력하게 됩니다.
MNIST data를 사용해서 한 번 학습해보겠습니다.
from tensorflow.keras.datasets import mnist (x_train, y_train), (x_test, y_test) = mnist.load_data() x_train = x_train / 255. x_test = x_test / 255. x_train = x_train[:,:,:,tf.newaxis] x_test = x_test[:,:,:,tf.newaxis] resnet = ResNet(10) resnet.compile(optimizer='adam', loss='sparse_categorical_crossentropy', metrics=['acc']) resnet.fit(x_train, y_train, epochs=5) resnet.evaluate(x_test, y_test)
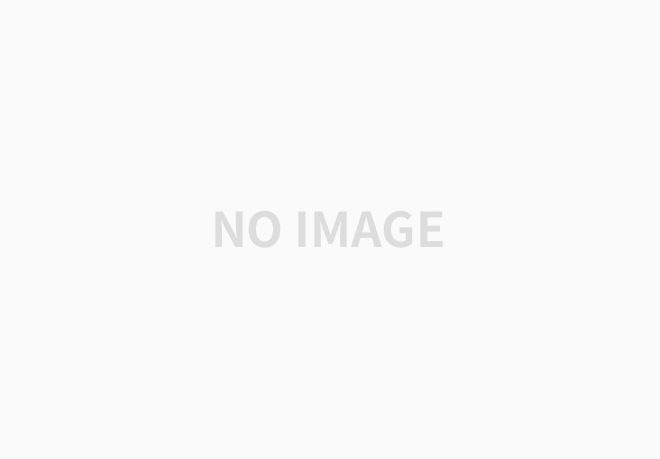
이전 게시글에서 간단한 MNIST model보다는 조금 더 좋은 결과를 보여주고 있습니다.
VGG Model 구현
Mini ResNet에 이어서 이번에는 VGG network를 구성해보도록 하겠습니다.(참고)
VGG network는 아래와 같은 형태로 구성되어 있습니다.
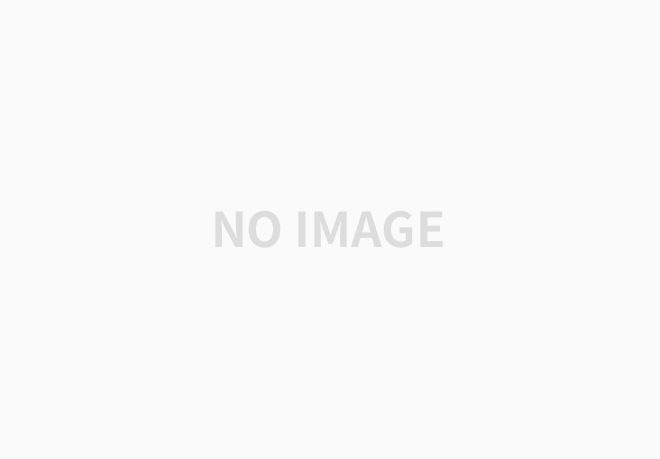
시작하기에 앞서서 model의 변수들은 __dict__와 vars를 통해서 접근이 가능합니다. 예를 몇가지 살펴보고 시작하도록 하겠습니다.
# Define a small class MyClass class MyClass: def __init__(self): # One class variable 'a' is set to 1 self.var1 = 1 # Create an object of type MyClass() my_obj = MyClass()
my_obj.__dict__
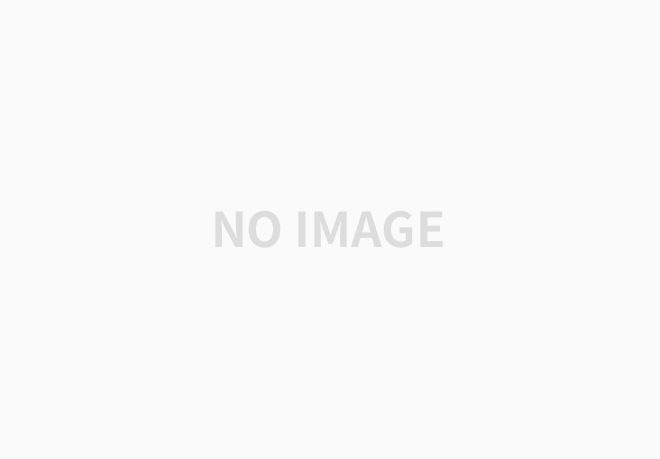
# Add a new instance variable and give it a value my_obj.var2 = 2 # Calls vars() again to see the object's instance variables vars(my_obj)
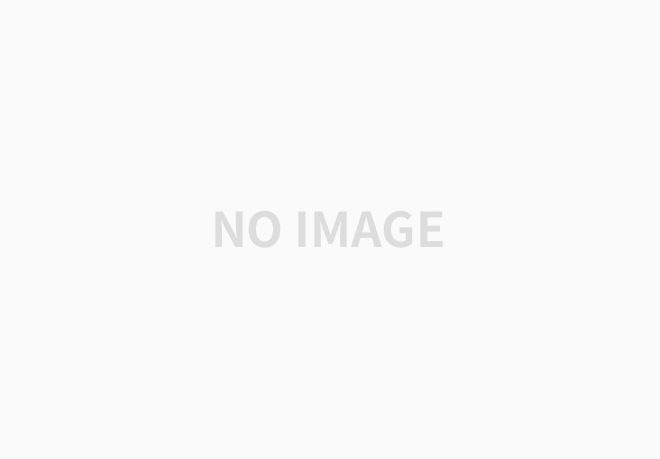
# Call vars, passing in the object. Then access the __dict__ dictionary using square brackets vars(my_obj)['var3'] = 3 # Call vars() to see the object's instance variables vars(my_obj)
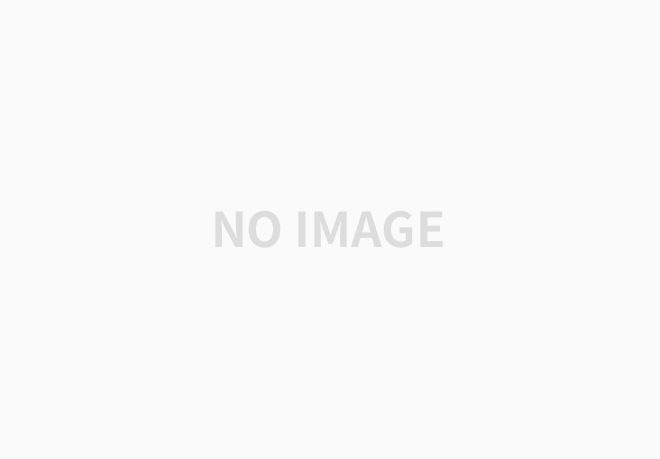
# Use a for loop to increment the index 'i' for i in range(4,10): # Format a string that is var vars(my_obj)[f'var{i}'] = 0 # View the object's instance variables! vars(my_obj)
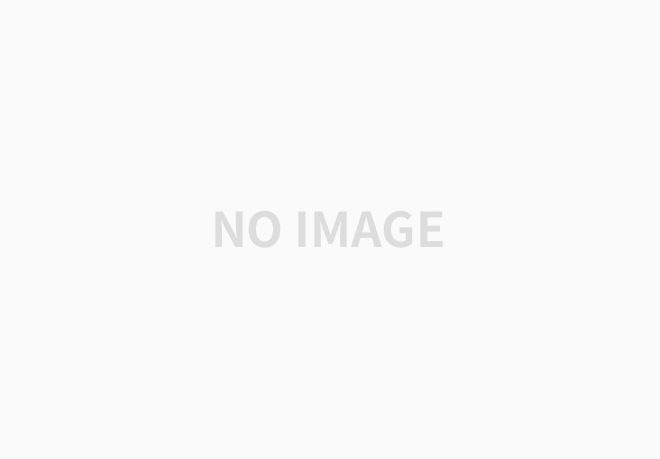
위와 같은 방법들을 사용해서 Block내의 layer에 접근하는 것에 유의하시기 바랍니다 !
VGG network는 몇 개의 Conv layer와 MaxPooling layer 조합의 Block이 여러개로 이루어진 network입니다.
먼저 이 Block을 생성할 수 있는 모델을 구현해보고, VGG network를 구현해보겠습니다.
class Block(tf.keras.Model): def __init__(self, filters, kernel_size, repetitions, pool_size=2, strides=2): super(Block, self).__init__() self.filters = filters self.kernel_size = kernel_size self.repetitions = repetitions # Define a conv2D_0, conv2D_1, etc based on the number of repetitions for i in range(self.repetitions): # Define a Conv2D layer, specifying filters, kernel_size, activation and padding. vars(self)[f'conv2D_{i}'] = tf.keras.layers.Conv2D(filters, kernel_size, activation='relu', padding='same') # Define the max pool layer that will be added after the Conv2D blocks self.max_pool = tf.keras.layers.MaxPool2D(pool_size, strides) def call(self, inputs): # access the class's conv2D_0 layer conv2D_0 = vars(self)['conv2D_0'] # Connect the conv2D_0 layer to inputs x = conv2D_0(inputs) # for the remaining conv2D_i layers from 1 to `repetitions` they will be connected to the previous layer for i in range(1, self.repetitions): # access conv2D_i by formatting the integer `i`. (hint: check how these were saved using `vars()` earlier) conv2D_i = vars(self)[f'conv2D_{i}'] # Use the conv2D_i and connect it to the previous layer x = conv2D_i(x) # Finally, add the max_pool layer max_pool = self.max_pool(x) return max_pool
VGG network를 이루는 block 조합 내 Conv layer의 반복 횟수 repetations와 filters 개수 등을 파라미터로 입력받고, Block을 구성하게 됩니다.
다음은 VGG network 입니다.
class MyVGG(tf.keras.Model): def __init__(self, num_classes): super(MyVGG, self).__init__() # Creating blocks of VGG with the following # (filters, kernel_size, repetitions) configurations self.block_a = Block(64, 3, 2) self.block_b = Block(128, 3, 2) self.block_c = Block(256, 3, 3) self.block_d = Block(512, 3, 3) self.block_e = Block(512, 3, 3) # Classification head # Define a Flatten layer self.flatten = tf.keras.layers.Flatten() # Create a Dense layer with 256 units and ReLU as the activation function self.fc = tf.keras.layers.Dense(256, activation='relu') # Finally add the softmax classifier using a Dense layer self.classifier = tf.keras.layers.Dense(num_classes, activation='softmax') def call(self, inputs): # Chain all the layers one after the other x = self.block_a(inputs) x = self.block_b(x) x = self.block_c(x) x = self.block_d(x) x = self.block_e(x) x = self.flatten(x) x = self.fc(x) x = self.classifier(x) return x
VGG network의 구현은 이렇게 이루어집니다. 총 5개의 block과 1개의 dense layer 그리고 최종 분류기(dense layer)로 구성됩니다.
- 참조
Coursera - Custom Models, Layers, and Loss Functions with TensorFlow : week 4
'ML & DL > tensorflow' 카테고리의 다른 글
[tensorflow] GradientTape (0) | 2021.01.12 |
---|---|
[tensorflow] Custom Training Loops (tf.GradientTape) (1) | 2021.01.12 |
[tensorflow] Custom Lambda layer / Custom layer (0) | 2021.01.11 |
[tensorflow] Custom Loss (Huber Loss, Contrastive Loss 구현) (0) | 2021.01.11 |
[tensorflow] Siamese Network (Fashion MNIST 비교 모델 구현) (0) | 2021.01.11 |
댓글